We have been optimizing the flow of electricity for charging electric vehicles from the cloud for years with our product “Gridware”.
In order to explore the possibilities of artificial intelligence in this regard in an application-oriented way, we joined forces with the University of Kassel and the House of Energy in the SALM (Self-Adaptive Charging Management) project. The results can be found here: SALM 2023 results booklet
Key points for us:
-
- The more complex the topologies and scenarios become, the more important the simulation.
-
- A simulation must be evaluated with metrics to compare different scenarios, topologies, and AI algorithms
-
- Visualization at the right level of abstraction is essential so that the operator understands what is actually happening
-
- The ramp-up of electromobility will only work to the extent that infrastructure development and user needs are in balance.
Next Step: We will use the findings to tailor Gridware even better to the needs of CPOs (Charge Point Operators):
-
- Metrics such as utilization, charging quality, energy per time interval will be made available at all hierarchy levels, i.e. for charging clusters, smart grids, campuses, areas, territories
-
- Algorithms are continuously improved in a measurable way
-
- In the future, we want to support our customers not only in the operation of charging infrastructure, but also in the design and electrical planning of the charging infrastructure. Build-out and expansion plans will be designed by simulating unlimited, sometimes multi-level wired AC and DC charging clusters at a site, on which different usage scenarios are then mapped. The scenarios include 1- and 3-phase vehicles with different battery sizes, charging states and feed-in acceptances. These occur at different times of the day, days of the week or other time intervals with some accumulation and are then comparable with each other.
-
- And yes: We are still missing an AI that works out and visualizes in which form the expansion of a topology should take place step by step, with regard to the expected scenarios… At the moment we still have to think along ;-).
Many thanks to the University of Kassel and the House of Energy for the successful cooperation!
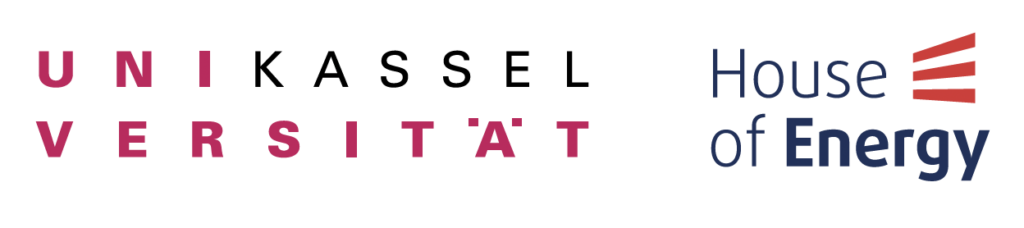